Inside Neuroscience: AI Models Map the Brain
Artificial intelligence is coming full circle. At the dawn of the field in the last century, scientists dreamed of mimicking human intelligence and the activity of the brain.
Now, AI is being used to study questions in neuroscience, including the operations of the brain itself.
The field was highlighted in November during a Neuroscience 2023 press conference titled, “The AI Edge: How New Tools are Advancing Neuroscience.”
Researchers at the event showed how they leverage machine learning, a branch of AI, for a range of biological tasks. Use cases included diagnosing depression, predicting Alzheimer’s disease, and determining where to place electrodes in the brain for deep brain stimulation.
Machine learning tools are trained on vast amounts of data. After training, they are tested and validated with new inputs to see if they can analyze data correctly.
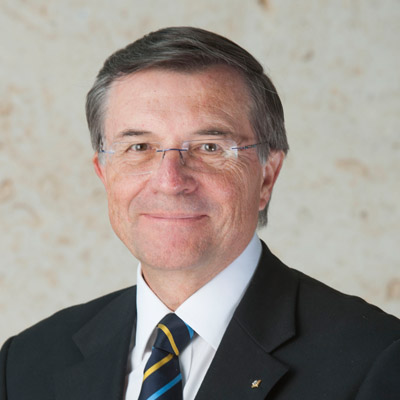
Terry Sejnowski
The idea is to create models that can “generalize” and assess data that they have never seen before, said moderator Terry Sejnowski, the Francis Crick Professor at the Salk Institute for Biological Studies and distinguished professor at the University of California, San Diego.
“This is very early days. It's like the first days when the Wright brothers got [an airplane] off the ground,” said Sejnowski of the field.
Diagnosing Disease
Machine learning models make sense of information too vast for a single human mind to grasp. Ayumu Yamashita leveraged this property to develop a diagnostic tool for depression, which is currently diagnosed through symptom assessment.
Yamashita presented a model to diagnose depression based instead on the underlying biology in the brain.
The model was developed using imaging data collected by functional MRI, which can assess the functional connectivity between brain regions. The number of potential connections exceeds 100,000, stymying efforts to use such data for classifying mental health.
But information is a boon for machine learning algorithms. And Yamashita and his colleagues used a lot of it to create their model. They pulled from an imaging dataset from 1,163 individuals, including 334 with depression.
They trained their model on fMRI data from people with depression and validated it with data collected from different imaging facilities.
Ayumu Yamashita
The model identified 83 connection patterns associated with depression, mainly between motor-related regions and the thalamus, which has a role in regulating emotion. Their approach outperformed conventional methods for linking fMRI data to depression, said Yamashita, an assistant professor at the University of Tokyo and visiting researcher at the Advanced Telecommunications Research International in Kyoto, Japan.
“Our study can improve the fundamental understanding of mental disorders and help in the development of new intervention methods,” he said.
Assessing Disease Risk
Researchers are also using machine learning to predict who will get disease. That’s a question that has long plagued the Alzheimer’s field.
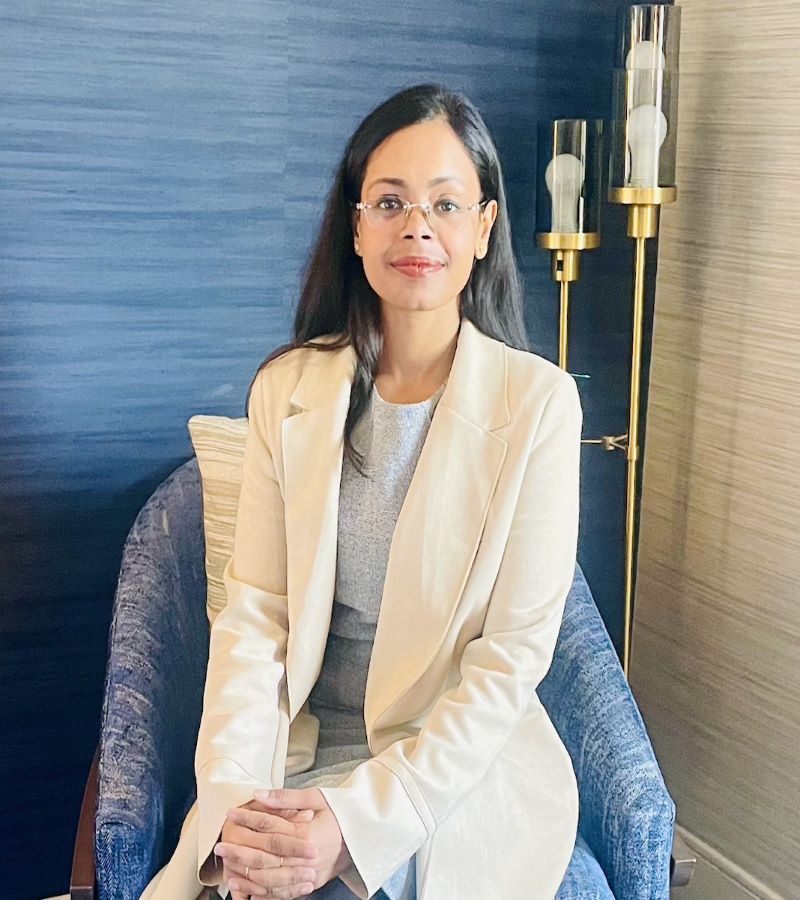
Nikita Goel
“The journey from normal aging to Alzheimer’s is not straightforward,” said Nikita Goel, a graduate student at the University of Southern California, at the event.
Before developing Alzheimer’s, people usually first transit through a stage called mild cognitive impairment (MCI). But not everyone with MCI progresses to Alzheimer’s. Goel and her colleagues generated a machine learning model designed to predict which individuals will undergo this transition.
They leveraged a dataset on 2,448 healthy people and people with MCI and Alzheimer’s, followed over years. The data contained information such as genetic risk, demographics, cognitive test results and brain imaging findings.
None of these factors alone are robust predictors of developing disease. But by combining them into their model, Goel and her colleagues found that they could better predict which individuals with MCI would go on to develop Alzheimer’s, and which healthy individuals would develop MCI.
The model may help in selecting patients for clinical trials that test how to stop progression of the disease, said Goel.
Augmenting Deep Brain Stimulation
Neural networks, a form of AI, are designed to more closely mimic human thought. Maral Kasiri and her colleagues are using neural networks to model brain connections, with the aim of predicting deep brain stimulation targets for children with dystonia, a movement disorder.
The process of implanting electrodes for the therapy requires two surgeries, and children often stay in the hospital a week. That’s because surgeons typically assess how the patient responds to stimuli in different brain regions before settling on the final implant location.
Kasiri and colleagues collected brain signals from children undergoing surgery and used the data to train a deep learning system to decode the signals. They then used their findings to build a model of the deep brain network. With the model, they could predict the response of the brain to stimulation in different regions.
The model enabled the development of an experimental tool to identify optimal brain targets for deep brain stimulation.
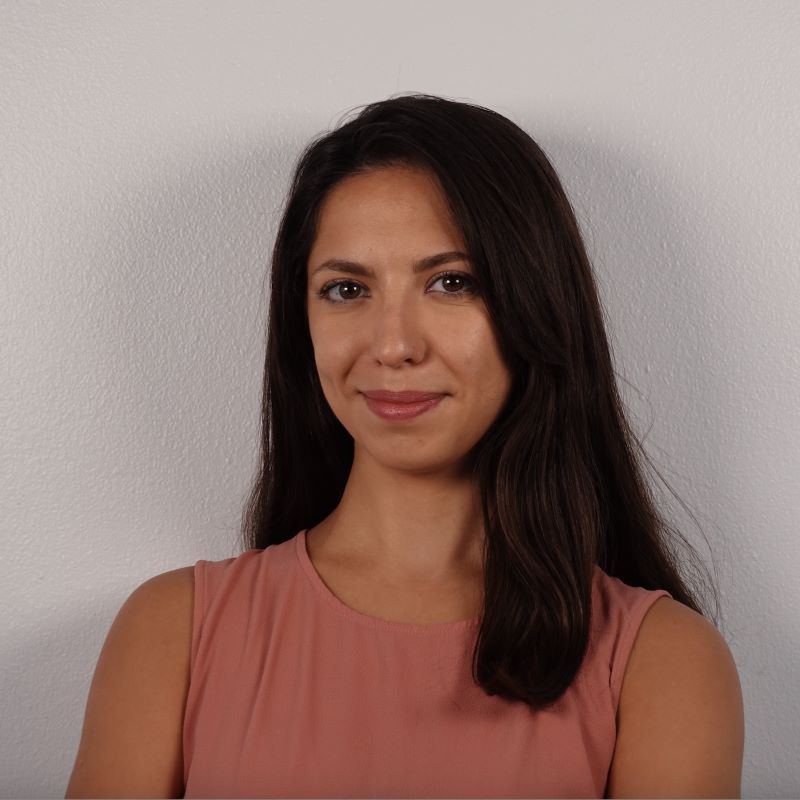
Maral Kasiri
“The ultimate goal is to reduce the time of the surgery and reduce the number of surgeries from two to one,” said Kasiri, a graduate student at the University of California, Irvine, of the preliminary findings. The approach may also be adaptable to similarly treated neurological conditions.
Edging Closer to Understanding Intelligence
An even more specialized type of AI, based on convolutional neural networks, is used to decode the brain circuits underlying vision. This type of neural network is good at tasks involving three-dimensional data and vision.
Dan Butts and his colleagues used the approach to model how cells in the brain’s visual cortex interpret light signals captured by the retina. To create their model, they fed it recordings from many neurons in a macaque presented with complex visual stimuli.
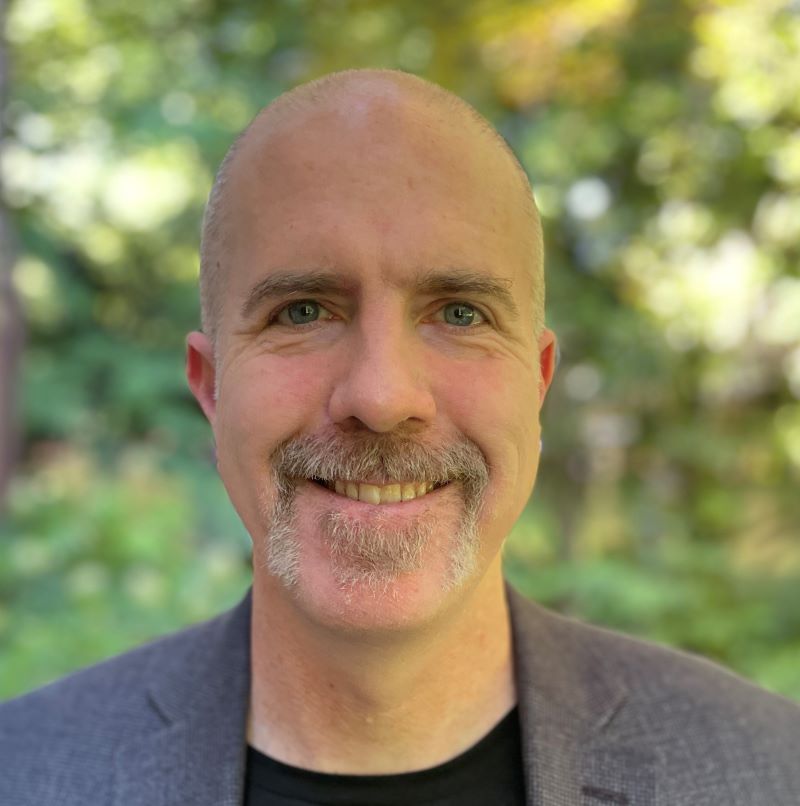
Dan Butts
The final model successfully traced the activity of a network of over 600 neurons, reported Butts, an associate professor at the University of Maryland. The researchers are now building a similar model with many times more neurons and studying complex visual tasks like high-acuity processing of color.
“A key barrier for understanding the neural basis of biological intelligence has been our lack of ability to interpret the electrical activity of the brain during sufficiently complex tasks,” said Butts.
Studying visual processing of complex visual stimuli is a step towards that larger goal.